I’ll demonstrate the following tasks:
- Download all zip files of FTDs
- Extract contents of zip file to .csvs
- Read + Combine zip files into dataframe. Apply filters (ie dates, tickers) during this process
- Chart resulting dataframe
Background information on FTDs and the site we will be using can be found below
- Definition / Background: https://www.investopedia.com/terms/f/failuretodeliver.asp
- SEC data: https://www.sec.gov/data/foiadocsfailsdatahtm
A copy of the notebook can be found here: Github
This shows the data/structure of the data and how the website is organized
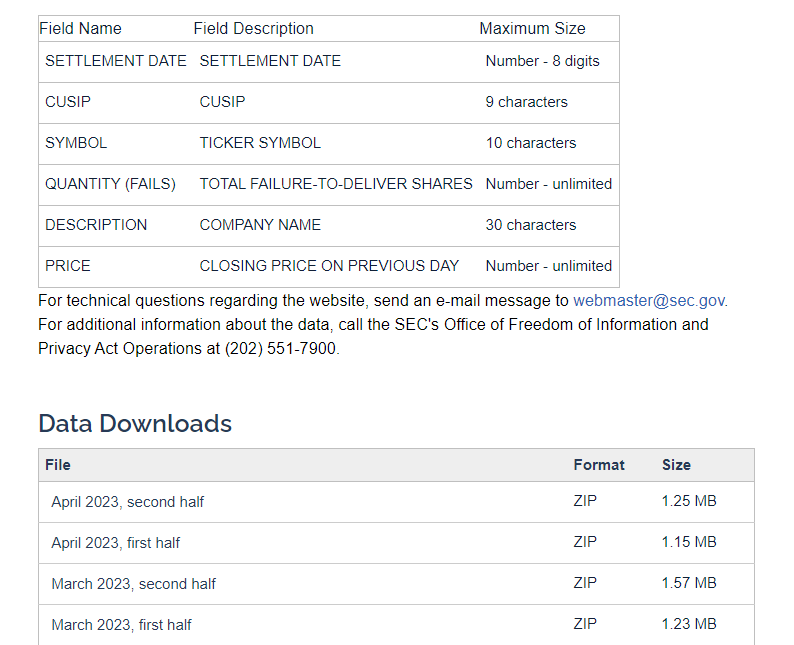
Download FTD Data
# Download every FTD Folder
import os
import requests
from urllib.parse import urljoin
from bs4 import BeautifulSoup
url = "https://www.sec.gov/data/foiadocsfailsdatahtm"
#If there is no such folder, the script will create one automatically
folder_location = r'C:\FTDs'
if not os.path.exists(folder_location):os.mkdir(folder_location)
response = requests.get(url)
soup= BeautifulSoup(response.text, "html.parser")
for link in soup.select("a[href$='.zip']"):
# for link in soup.find_all('a'):
#Name the pdf files using the last portion of each link which are unique in this case
filename = os.path.join(folder_location,link['href'].split('/')[-1])
# print (filename)
#check to see if file exists or not before saving
if os.path.isfile(filename):
print (filename + "already exists")
else:
with open(filename, 'wb') as f:
f.write(requests.get(urljoin(url,link['href'])).content)
Open Each Zipped Folder & Extract
import zipfile, os
working_directory = folder_location
os.chdir(working_directory)
for file in os.listdir(working_directory): # get the list of files
if zipfile.is_zipfile(file): # if it is a zipfile, extract it
with zipfile.ZipFile(file) as item: # treat the file as a zip
item.extractall() # extract it in the working directory
Add Each Extracted File To A Dataframe
# importing the library
import os
import pandas as pd
# control parameters for below. Change here as needed
startPeriod = 20200101 # YYYYMMDD
tickerList = ["AAPL"]
# giving directory name
dirname = folder_location
# giving file extension
ext = ('.txt')
# iterating over all files
df_final = pd.DataFrame(columns=['SETTLEMENT DATE','CUSIP','SYMBOL','QUANTITY (FAILS)',
'DESCRIPTION','PRICE'])
for files in os.listdir(dirname):
if files.endswith(ext):
# print(files) # printing file name of desired extension
# print(dirname + "/" + files)
# print(files.Path())
# print(dirname+files)
# df = pd.read_csv(dirname + "/" + files)
# df.head()
df = pd.read_csv(dirname + "/" + files,sep='|', encoding='ISO-8859-1',on_bad_lines='skip')
# only run for certain years
sub_df=int(df.iloc[0]['SETTLEMENT DATE'])
if sub_df>=startPeriod: #YYYYMMDD
df = df[df['SYMBOL'].isin(tickerList)] #Filter by ticker symbol
# print (df)
df_final = pd.concat([df_final, df],join="inner")
else:
continue
print ("Done!")
Review The Dataframe
Note that price in this exploration is listed as an object. We need to convert this to a number for charting purposes later.
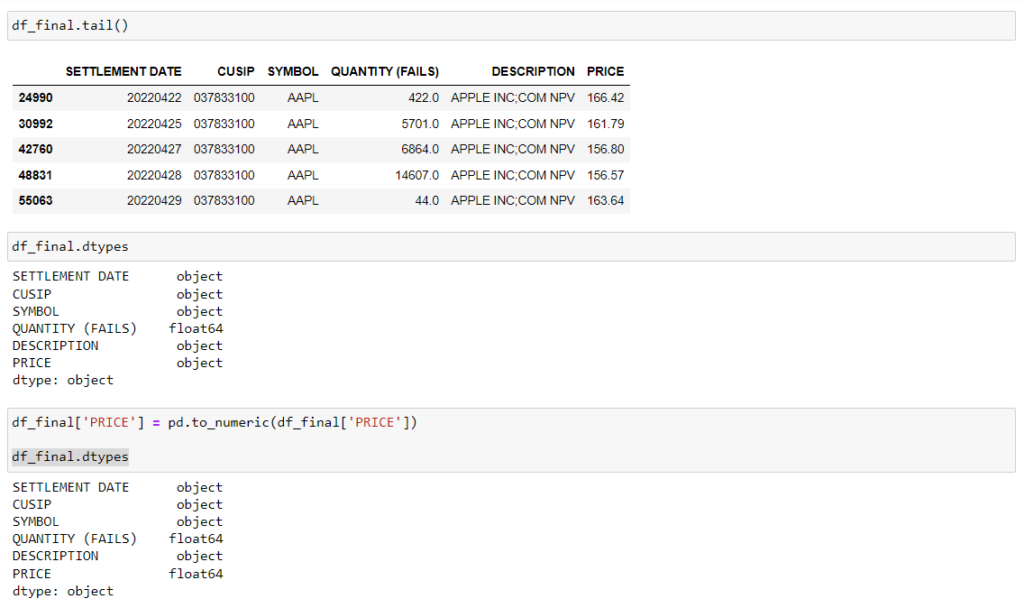
Chart
import matplotlib.pyplot as plt
# df = pd.read_csv("review_ftd.csv")
df = df_final
fig, ax = plt.subplots(figsize=(20,10))
df.plot(x = 'SETTLEMENT DATE', y = 'QUANTITY (FAILS)', ax = ax)
df.plot(x = 'SETTLEMENT DATE', y = 'PRICE', ax = ax, secondary_y = True)
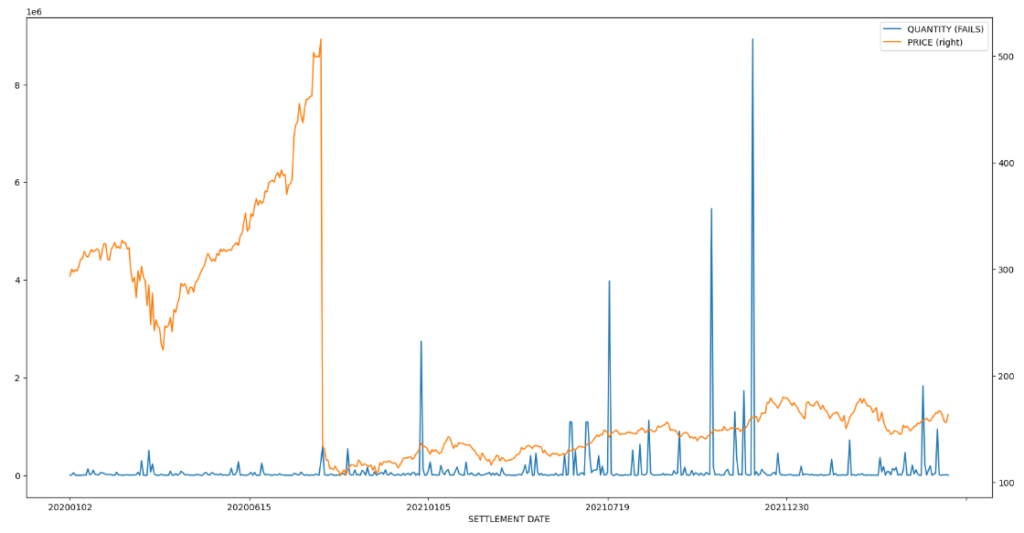